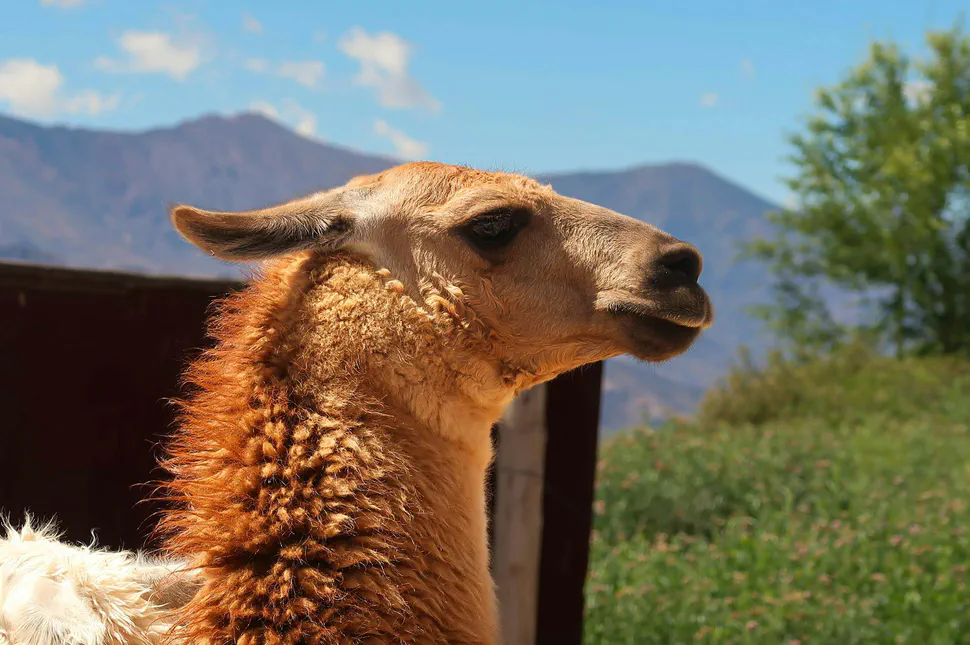
What is worth of your data to LLM?
When a machine learning model is trained on a dataset, not all data points contribute equally to the …
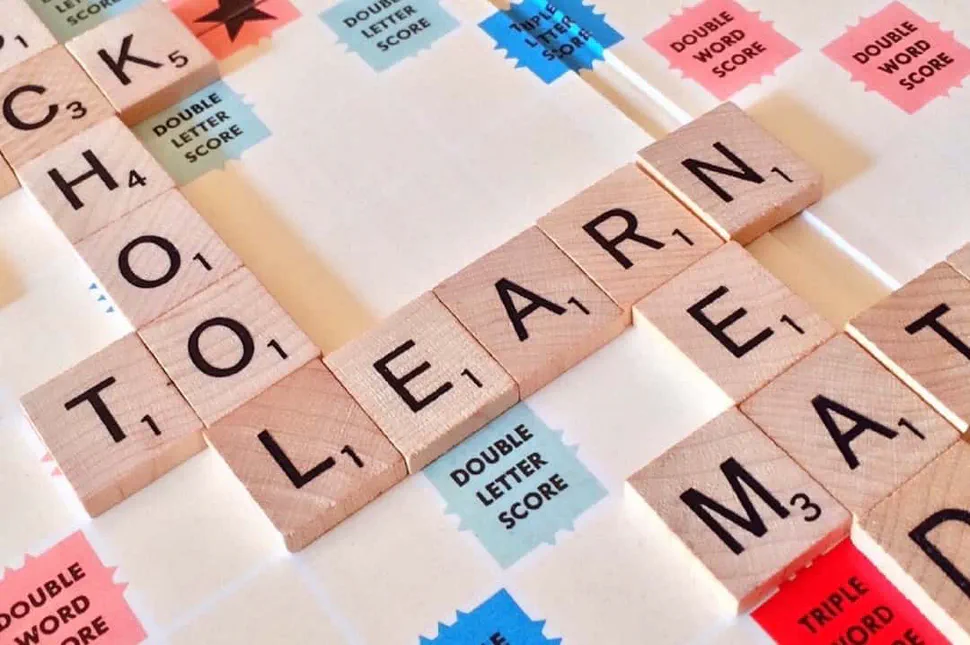
How machine learning is accelerating data integration?
Data integration generally requires in-depth domain knowledge, a strong understanding of data …
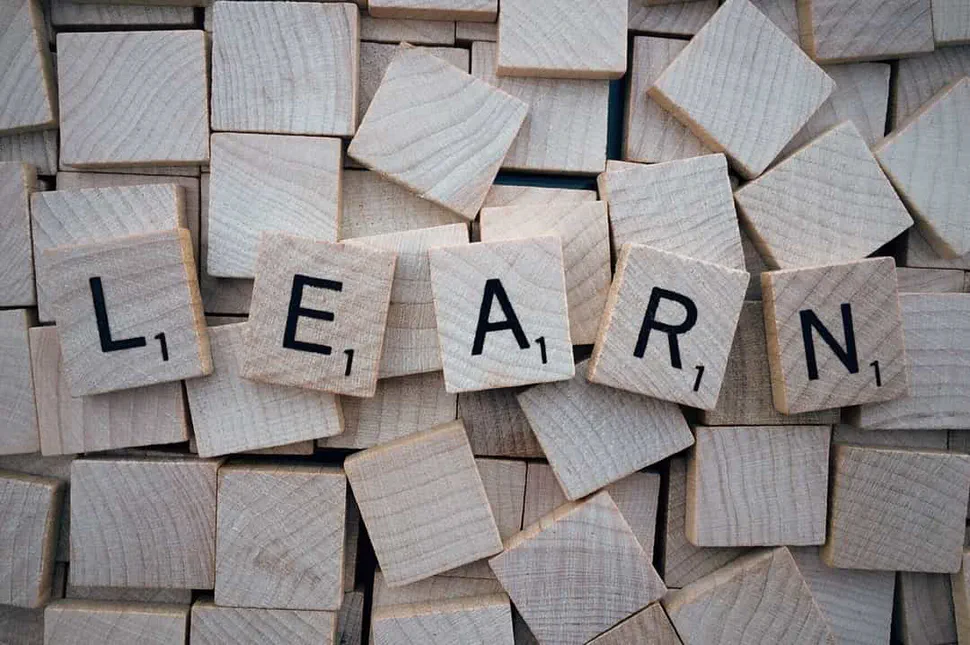
Upcoming of the learned data structures
Can machine learning-based data structures i.e. learned data structures replace traditional data …
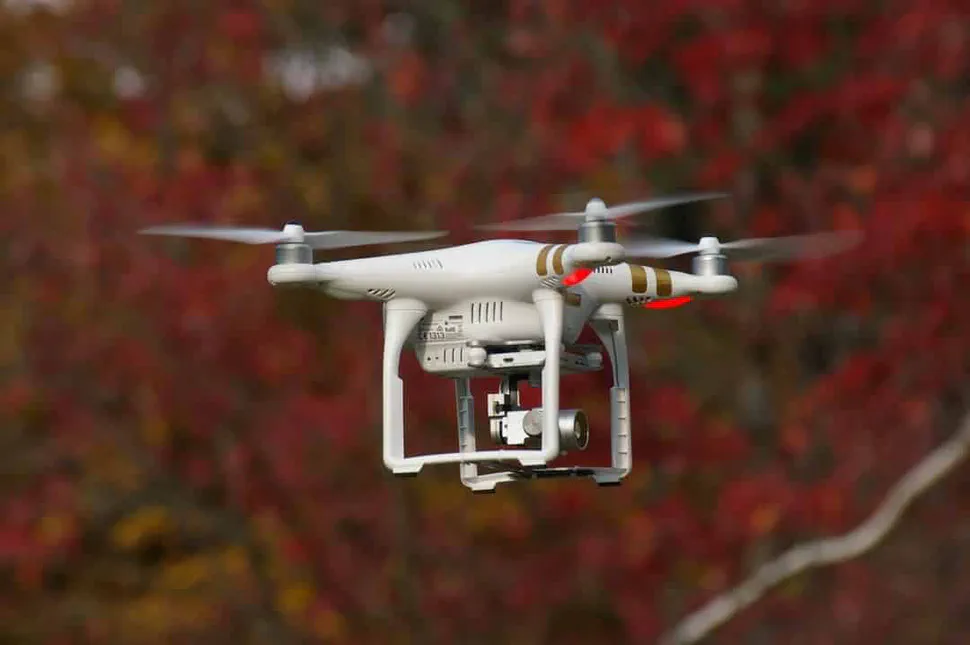
Bias and Fairness in Machine Learning
In AI and machine learning, the future resembles the past and bias refers to prior information. …
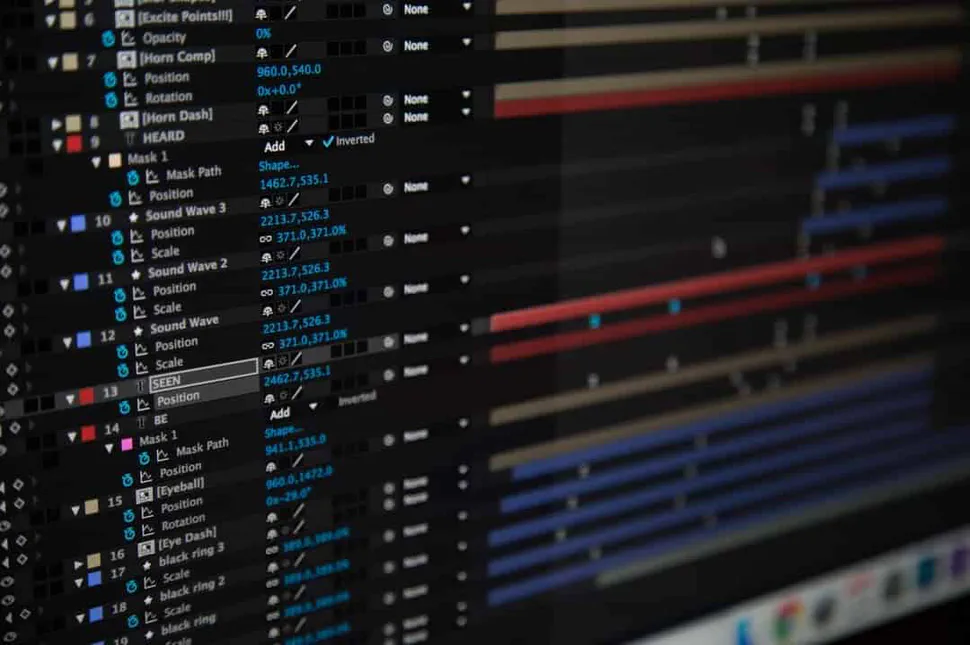
What it means for a machine to learn or think like a person?
In a recent article, Lake et al.1 examine what it means for a machine to learn or think like a …